Tim's Portfolio
Acoustic Reconstruction using Synthetic Aperture Focusing
Tim Straubinger - October 2021
MSc. Thesis
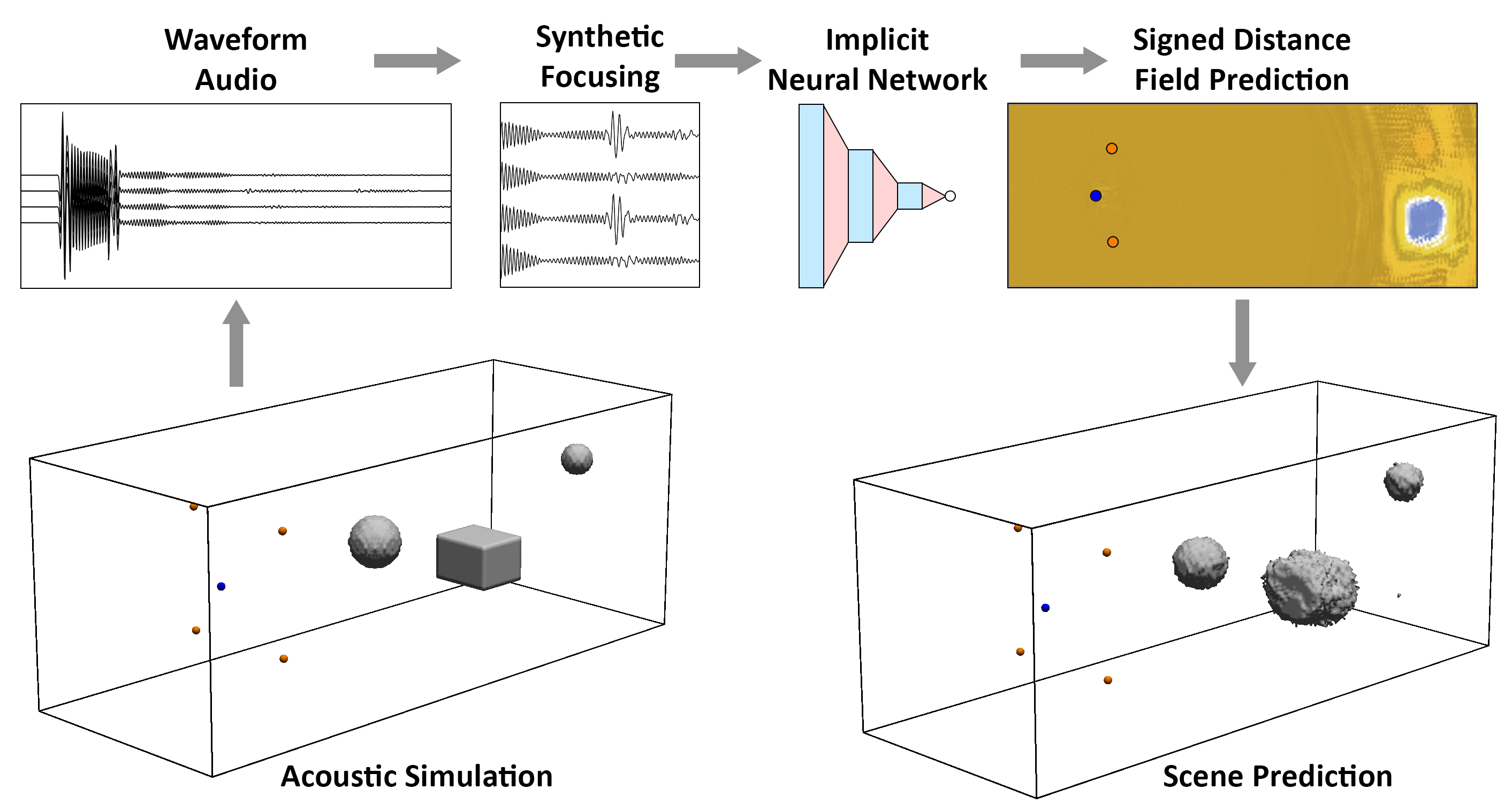
Navigating and sensing the world through echolocation in air is an innate ability in many animals for which analogous human technologies remain rudimentary. Many engineered approaches to acoustic reconstruction have been devised which typically require unwieldy equipment and a lengthy measurement process, and are largely not applicable in air or in everyday human environments. Recent learning-based approaches to single-emission in-air acoustic reconstruction use simplified hardware and an experimentally-acquired dataset of echoes and the geometry that produced them to train models to predict novel geometry from similar but previously-unheard echoes. However, these learned approaches use spatially-dense representations and attempt to predict an entire scene all at once. Doing so requires a tremendous abundance of training examples in order to learn a model that generalizes, which leaves these techniques vulnerable to over-fitting.
We introduce an implicit representation for learned in-air acoustic reconstruction inspired by synthetic aperture focusing techniques. Our method trains a neural network to relate the coherency of multiple spatially-separated echo signals, after accounting for the expected time-of-flight along a straight-line path, to the presence or absence of an acoustically reflective object at any sampling location. Additionally, we use signed distance fields to represent geometric predictions which provide a better-behaved training signal and allow for efficient 3D rendering. Using acoustic wave simulation, we show that our method yields better generalization and behaves more intuitively than competing methods while requiring only a small fraction of the amount of training data.